Once a patent is filed, it is out there in the open for everyone to see. However, many companies wish to keep their important inventions hidden. So at times, they do this by performing conscious mistakes in the patent document. Additionally, clerical or database error also eliminates important information in the patent. When companies request patent searches, they opt for automatic data Cleaning and Validation methods to save money and time. This is where such clerical mistakes go unnoticed because of set filters. Analysts easily miss the important breakthrough inventions that could change the game.
If patent research is your bread and butter, you already know how important data cleaning and validation are to produce statistically relevant results. Given the time it consumes, automated processes have come to light for data cleaning during patent landscape projects. However, “let’s do it in less time and save money” isn’t the attitude to get promising results and thus doesn’t align with GreyB’s way of, ‘if it exists, we’ll find it.’
Artificial intelligence improvement has made companies believe that the manual effort in data cleaning can be ignored. But, it cannot be overlooked because the accuracy of the automatic method is only as good as the algorithm used or the algorithm developer’s knowledge of facts.
Additionally, automatic methods won’t pick up clerical mistakes in the patents. Hence, until and unless patents are errorless, automatic data cleaning systems cannot be completely relied on.
Since we come across a large amount of patent data, we, as analysts, perform a manual data cleaning & validation part.
In theory and In practice
The examples below showcase why data cleaning & validation cannot be completely accurate until checked manually and why we prefer it for all of our clients.
Clerical mistakes present in the Assignee data.
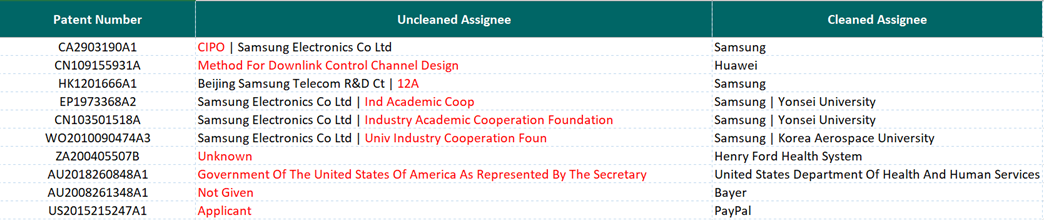
It is worth noting that the above mistakes are just a few examples, and as long as the data is left unattended without manual cleaning, they are bound to happen.
- The first mistake we usually see is that when two companies are listed in the uncleaned assignee, it denotes a possible collaboration. But, when cleaned manually, we often see no collaboration, and only one company is involved. Like in the patent number CA2903190A1 CIPO is not an assignee name, but in actuality, it is the name of the Canadian Intellectual Property Office. Also, in patent number HK1201666A1, 12A can appear in automatic data cleaning but it is just junk data.
- As discussed in the beginning, the actual assignee’s name may sometimes be hidden. For example, patent number CN109155931A has Assignee Data which has nothing to do with the company’s actual name. You can easily miss such things by running the relevancy check through artificial intelligence tools.
Moreover, if you fall prey to these mistakes, you will miss the big names working on new and unique technology in your report. We believe a few hours of additional effort is worth it and hence cannot be overlooked.
Types of Clerical mistakes in Inventor data
The clerical mistakes further extend to the uncleaned inventor data as well. Again, these are just a few examples, and it is impossible to list exact clerical mistakes. These mistakes remain unique to every patent without a set pattern.
Let us show how they can impact our analysis if not handled efficiently.
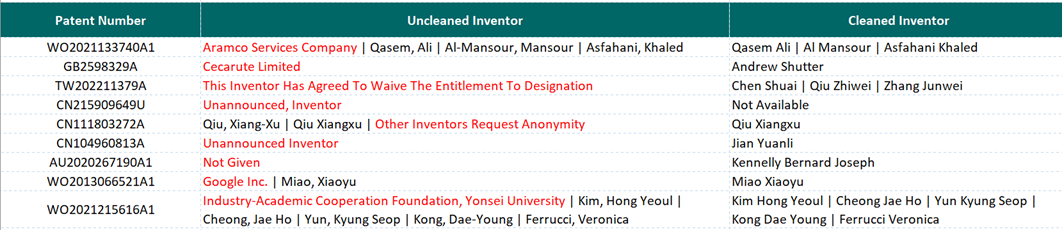
As you can see in the patents GB2598329A, CN104960813A, and AU2020267190A1, the raw data denotes no inventor name. This same detail will be picked up by an automatic tool. As a result, your report won’t be satisfactory. But, if the patent is closely studied, the inventor’s names will come to light. You may ask how we follow the manual method to get accurate results. Let us explain it through an example.
For patent number AU2020267190A1, Inventor’s name is not given,, but when we study the patent, another family member is attached to this invention. That family member is a WIPO application; when we study the WIPO application, inventor name details can be extracted as it is common to all family members. Imagine if this patent went through an automatic database, all these details would swoop off from right under our noses.
Since clients trust us, they don’t want haphazard results, and that too when they are paying for this work and hoping to catch some good leads.
Now, all the above examples represent a scenario where missing some hours of manual work will give a report that is nothing but uncleaned data with a facade.
So are we saying that dealing with the uncleaned data above is enough? To attain accurate results from data cleaning and validation, it is important to understand those data problems exist in every single field, like dates, abstracts, claims, or even patent images. Below are some examples to explain such cases in detail.
Types of mistakes in Patents images data
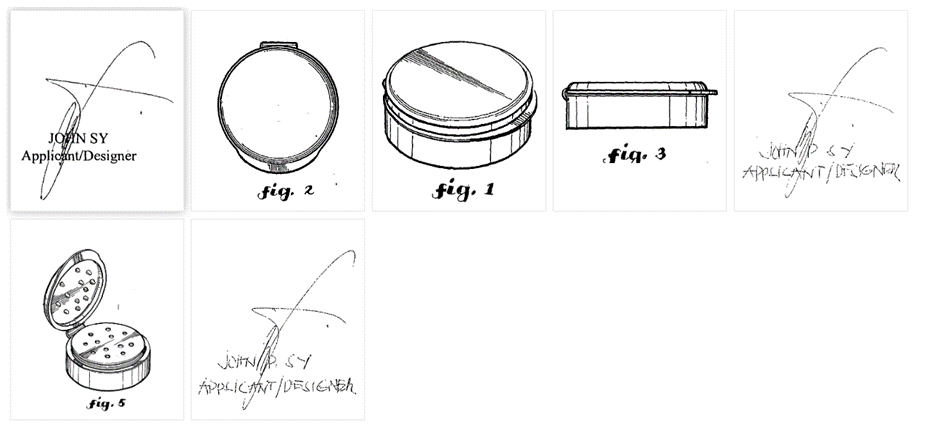
Given above are various images collected from the patent through an automatic tool. The tool even considered the signature of the applicant/designer of value, but in actuality, only Fig 2, Fig 1, Fig 3, and Fig 5 are of interest.
The patent images are of high value when we work with clients who especially require the design of patent images. Moreover, in such cases, the textual data is not available.
While the images in the raw form won’t make much sense in the report, we must deduce the key points through them for the client.
Manually, data cleaning and validation in the case of patent images involves reviewing every single patent data to provide junk-free patent images not only from databases that provide the same but for those patents where the images exist only in original patent documents.
Further, translation takes up most of the time in data cleaning because ignoring the same in the claim part can become problematic. Below is one example where any such special character can be a major problem for readers/clients.
Types of translation issues in claims data
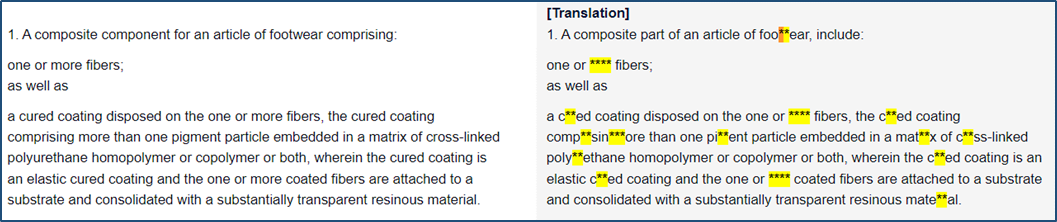
The example highlighted above is a typical case where the translation replaced important words in the claim with characters. If the translation is passed to the client without a manual go-through, how badly will it impact his trust in the service?
Thus, GreyB Analysts rely more on their learnings than on automatical tools. Ultimately, the client deserves the best results and value for money.
Conclusion
To err is human.
Until patents are handled manually, errors will appear in the uncleaned data. The only way to tackle this error is to handle it manually too. At GreyB, we ensure that our team manually cleans and validates the data before delivering any report. This is how we to deliver results that can be relied upon. We invest more time into the projects to provide value for the client’s money. If you wish to experience the change manual data cleaning and validation can make, simply
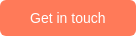
Authored by: Sachin Singh, Data Extraction and ETL
Edited By: Nidhi, Marketing
Next Read: How to Manage your Organization’s Data efficiently?